The Power and Pitfalls of AI in Business: Navigating the Maze of Decisions and Transparency
By Kilian Pfahl *
AI-Focused Investigative Procedure in Business Operations
The emergence of Large Language Models (LLMs) like ChatGPT is revolutionizing business operations. These models expedite data processing and generate precise answers, offering companies valuable opportunities. However, they also carry substantial legal and operational risks, particularly in the realm of corporate due diligence. Business leaders are left pondering: How do we responsibly utilize this technology? Can we truly trust its applications? And does corporate due diligence compel us to incorporate LLMs in our operational processes?
Key Role in Mergers and Acquisitions (M&A)
LLMs boast a wide array of applications, particularly in areas with high legal requirements and data-intensive processes. Notably, in the context of M&A transactions, these models can play a significant role by dramatically accelerating the due diligence process. They enable swift analysis of extensive documents and help detect critical contractual clauses or risks early on, resulting in an efficient, less error-prone review phase and a more informed decision-making basis.
LLMs also excel in contract management, identifying potentially ineffective clauses and suggesting necessary modifications. In areas like non-compete agreements or liability limitations, they help ensure contracts are legally secure and ward off strategic blunders. Furthermore, they find application in compliance monitoring, assisting companies in keeping pace with rapidly changing legal landscapes like data protection or financial regulation, facilitating swift implementation of necessary adjustments.
The Illusion of Competence
Despite LLMs' potential, their accompanying risks must not be trivialised. Business leaders must acknowledge the technology's weaknesses to steer clear of errors and liability pitfalls. It's essential to understand that LLMs are not "super AI." They are sophisticated text generators that mimic intelligence by predicting the most likely sequence of words based on vast datasets. While they simulate intelligence by calculating which word is most likely to follow the previous word, the results offer answers that may seem plausible but are not always accurate.
LLMs lack human-like understanding or knowledge, and they cannot validate content or engage in deep cognitive thinking. Their strength lies in their ability to recognize statistical relationships between words, which they implement in coherent text. Nevertheless, the result is always a product of probability calculations, with no guarantee of accuracy. Thus, leaders should never presume LLMs offer a comprehensive or infallible solution, especially in critical, legally relevant areas. LLMs should be utilized only as supportive tools and not as the lone basis for decision-making. Relying solely on LLMs or unskilled personnel might be considered negligent due diligence.
Another hazard concerns the lack of transparency in decision-making. LLMs often function as black-box models, making their inner workings and decision-making logic challenging for users to grasp. However, businesses must legally base decisions on traceable, verifiable information. The Business Judgement Rule (§ 93 Abs. 1 AktG) demands that entrepreneurial decisions be both transparent and well-founded. Without this transparency, liability claims might ensue, as insufficiently documented decision-making may be viewed as a breach of duty of care.
Moreover, results may be skewed by bias (preconceived notions) within the training data of LLMs. These models are trained on vast datasets that often harbour historical biases or imbalanced representations. These biases may inadvertently transfer into generated answers, leading to erroneous or discriminatory decisions. Should nine out of ten training datasets be factually incorrect or lacking scientific foundations, the tenth dataset might be unjustly disregarded, despite offering valuable, reliable information. The AI output could thus be flawed.
Liability and Duty of Care
The liability of business leaders in relation to the use of LLMs is one of the core legal challenges. Under the Business Judgement Rule, liability is waived if decisions are made based upon fitting data and with required care. The decision-making process must be rooted in sound, verifiable data. When employing LLMs, the question arises as to whether these results satisfy this premise. The "illusion of competence" described above could lead business leaders to make decisions based on seemingly compelling but ultimately erroneous data.
Therefore, leaders should ensure that LLMs are used as supplementary tools and not the exclusive basis for decisions. A decision solely based on AI-generated content could be construed as a breach of duty of care.
Documentation Dilemma
Another challenge is the documentation of decision-making. When liability issues arise, business leaders must demonstrate that their decisions were grounded in solid, traceable information. The opaque nature of LLMs poses difficulties in this regard. A court may view the absence of transparency as a breach of duty of care.
Furthermore, there is the "Caremark Duty": Business leaders must establish suitable monitoring mechanisms to identify and address risks promptly. If LLMs are deployed for risk management, the systems used must be transparent and reliable. This includes managing potential risks such as bias or misinterpretations. The lack of control over or traceability of AI decisions could pose complications in liability matters.
Towards Responsible Use
Business leaders confront the challenge of leveraging LLMs effectively while acting responsibly with regard to due care. The judicious deployment of these technologies is an integral component of overall decision-making, which inevitably requires human judgment (as long as present-day technological standards persist). In specific situations, AI integration might not be an option but a requirement, provided it demonstrably enhances decision-making efficiency and quality. However, this necessitates clear governance, defined processes, and employee training to comply with legal requirements and minimize associated risks. Only then can AI be employed responsibly and consonant with the duty of care.
Dr. Kilian Pfahl is a Senior Associate at Hogan Lovells.
Enrichment Data:
Data Protection & Privacy Compliance
- Anonymization & encryption: To prevent unintended release of PII, sensitive data in training sets and queries should be anonymized and encrypted[1][5]. Implement stringent access controls and logs for LLM interactions.
- Compliance alignment: Comply with GDPR and other regulations by restricting data retention periods and executing Data Protection Impact Assessments (DPIAs), as per the EU's guidance on LLM privacy risks[1][4].
- Supply chain audits: Scrutinize third-party LLM providers for their security practices, to forestall data compromises via insecure APIs or datasets[5].
Governance & Risk Frameworks
- Risk taxonomy adoption: Apply frameworks like OWASP’s LLM Top 10 (e.g., LLM08 for excessive agency) to detect threats such as unauthorized financial transactions or biased outputs[5].
- Geopolitical vetting: Steer clear of LLMs developed in jurisdictions with conflicting data laws or ideological agendas to mitigate bias risks in sensitive areas like voting guidance[3].
- Continuous monitoring: Deploy AI-specific threat detection systems to detect prompt injection attacks or unusual behaviour in real time[2].
Operational Safeguards
- Purpose limitation: Constrain LLMs to narrowly defined tasks (e.g., document summarization) to minimize "hallucination" risks and unauthorized actions[5].
- Human oversight: Implement human review loops for significant outputs, including contract analysis and compliance reports.
- Transparency protocols: Make public LLM usage and document decision-making processes, to meet regulatory expectations[1][4].
Due Diligence Use Cases with Mitigations
| Application | Risks | Mitigation ||--------------------------|-------------------------------------|-----------------------------------------------------------------------------------------------------|| Contract review | Data leaks, misinterpretation | Employ local LLM deployment with redaction tools; institute human validation for crucial clauses || Entity screening | Bias in risk scoring | Cross-check outputs with multiple LLMs and conventional databases[3] || Market analysis | Propagation of misinformation | Cite sources with links to original documents; implement fact-checking pipelines |
By incorporating these measures, businesses can capitalize on LLMs for rapid data processing in due diligence while preserving compliance and operational integrity.
- In business, Large Language Models (LLMs) like ChatGPT, although revolutionizing data processing and decision-making, carry significant legal and operational risks, especially in due diligence.
- Whether in mergers and acquisitions, contract management, or compliance monitoring, LLMs can accelerate and improve the efficiency of various processes but should not be the sole basis for decision-making to avoiderrors and potential liability.
- Business leaders should be aware that LLMs, despite their advanced capabilities, don't possess human-like understanding or knowledge and can't validate content or engage in deep cognitive thinking. Their results are based on probability calculations, with no guarantee of accuracy.
- The lack of transparency and the susceptibility to biases within LLMs raise concerns for traceable and verifiable decision-making, which is essential to meet the Business Judgement Rule requirements.
- To navigate the risk landscape, businesses should adhere to data protection and privacy guidelines, risk taxonomy adoption, governance and risk frameworks, operational safeguards, and due diligence use cases with mitigations, ensuring AI integration while preserving compliance and operational integrity.
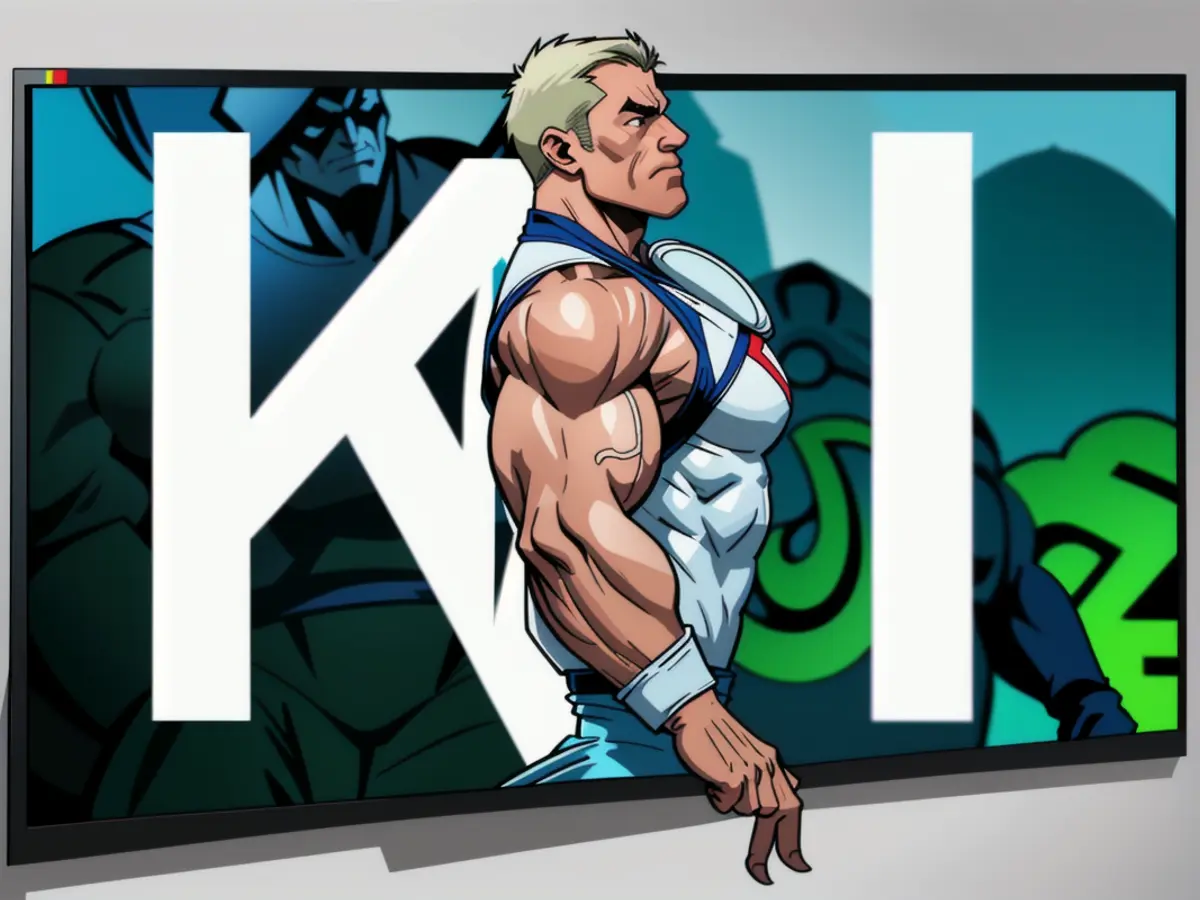